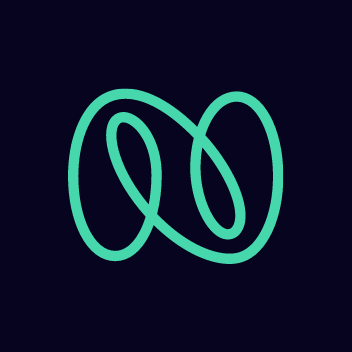
Nagarro
Predictive maintenance is straightforward when it comes to being defined but proves difficult to address when it comes to building an IoT Analytics solution for it. As a result, Proof of Concept projects are always the start for such an implementation but also the point when you start asking:
Where does the complexity come from?
Why are decision makers asking for proofs of concept (PoCs) before being convinced that investing in an analytics solution is the right choice?
Is predictive maintenance as easy to define as it looks at first sight?
Are all problems structured well enough so that data-driven solutions can be easily implemented?
How much of the problem components are technical details, process specific or business context dependent?
The context for a predictive maintenance PoC
All the above are not just valid questions for decision makers, but also translate into cost generators and efficiency controllers that must be considered and well balanced for a comprehensive solution. Predictive maintenance challenges are usually highly specific to industrial machinery and can be summarized by two instances:
- Highlighting the functional disorders of machines, thus predicting risk of failure
- Monitoring the normal functionality but benchmarking against predefined events such as human intervention or process requirements, thus preventing operational loss.
Given this, the need for a Proof of Concept before stepping into large-scale implementation comes as a natural first step. However, the risk is that once you have a well-defined concept that addresses most dependencies for a predictive maintenance issue, the complexity regarding business, people, processes, and sometimes data limitations, becomes visible. In such cases, a business decision maker will be tempted to reassess the entire context, leading to delays or even a “No Go” for moving from proof of concept to implementation.
Must-have perspectives
Once the predictive maintenance problem is well defined in a non-technical description, clearly stating the expected outputs, the PoC is ready to fly out. A viable PoC should offer answers or at least create awareness from the following angles:
- Data perspective — Data completeness is the foundation of any conceptualization of the predictive maintenance problem. This means many things, but essentially it refers to mapping all processes and events that characterize the maintenance instance against its specific data stream. Having this covered, analyzing, modeling and visualizing the results become almost an automatic exercise.
- Technical angle — This is in a way related to the above-mentioned data requirements but is more of an engineering perspective. Even if we refer to hardware connectivity, data collection and transformation technology or analytical software engines, all these must work together as a whole. Eventually, such disruption might be a hard barrier for large-scale implementation, so it is mandatory to be explored during a PoC.
- Process constraints — Besides the effort of gathering the proper data for the right purpose, aligning all technical requirements and relevant modeling technologies, there are operational processes involved that must be considered because the maintenance problem is always subject to a controlled environment. Usually, this translates into rules and constraints that cannot be avoided. It is the mission of the PoC to capture ALL as such. Even if a few of these process constraints are missing, can set the larger implementation to failure.
What’s next?
After the PoC has proven its utility by solving the use case, a more significant problem arises: integrating the IoT solution for a chosen situation into the bigger operational context. This usually is a sparser universe than generally expected and accepted.
Even if an IoT implementation is about data, modelling and programming, one should not forget that the usage and decision-making still belongs to humans and, like any service provided, it must have a business justification translated into profitability. These two are enough to create further challenges for large-scale implementation after a successful PoC.
We refer to hidden dependencies, which become visible only after the conceptualization phase when a broader context is discussed. Below are just two examples reflecting this reality.
Technological differences are difficult to bridge
We faced many situations where predictive maintenance was intended to be adopted through IoT solutions for manufacturing machinery as part of production processes. The PoC was about collecting streaming data from smart machinery equipped with sensors of all kinds, selecting monitoring KPIs, building machine learning algorithms for abnormal functionality pattern recognition and triggering alarms for operational decision makers.
While everyone from the board of directors accepted the evidence that such an approach will bring tremendous optimization for the production line, there was a severe constraint for continuing the PoC with a large-scale implementation: the technological differences in machinery across various operational partners across the globe. Without having this alignment, the solution was impossible to scale up and having technological consistency across all partners was a considerable investment to make. So, the barrier, in this case, was the strategic decision of investing in technological alignment before adopting an IoT data-driven predictive maintenance practice.
Cultural adoption of automated processes
Referring to the industry of consumer goods manufacturing, the reality is that many of the Quality Control processes are still human-based and quality data collection still relies on human input. Even if at first glance this process would not fall into a predictive maintenance IoT PoC, the truth is that given the explosion of sensors and image recognition algorithms, defect recognition, which is a QA task, can be automated with ease. It is not hard to understand that having this process automated and having the capability of real-time defect detection, predictive maintenance is just a matter of going back to the root cause when such instances are identified.
We faced situations where after having a successful PoC on QA IoT automation, the transfer from human assessment to humans just monitoring the IoT QA process was a challenge. Cultural differences, even in between different departments of the same organization required intensive skills upgrade and even professional reconversion.
So, even having a successful PoC, the decision for moving to large-scale integration and adoption was delayed due to impact on a specific category of employees. If it’s about to go back to PoC design, it is not unusual to include a UI design into the proposed solution for replicating the current QA process as close as possible or to conduct feasibility studies for evaluating collateral dependencies.
Conclusion
Finally, IoT Analytics is still a treasure trove of solutions to the challenges of predictive maintenance. Most manufacturing industries, and not only, are feeling more and more the need for automating their production and QA processes. The human role in production environments is changing towards a more supervising and decision-making role, data collection and storage is possible without any restrictions. Predictive algorithms are naturalized into IoT platforms to the extent that it is just a matter of making the right selection for the predictive model and no longer a state of the art.
What is left, is shifting to a data-driven culture, which requires significant investment with dependencies and implications for the entire business ecosystem. For this, service providers such as Nagarro can offer support for building a data-driven culture maturity curve — a map that will guide you forward.