An average person makes 35,000 decisions per day (with around 288 decisions solely about food!). If these are the numbers for an average individual, imagine the decision-making scale involved when talking about business organizations. Organizations must make critical decisions every day, and these decisions are only becoming more complex.
A recent Gartner survey suggests that effective decision-making keeps getting more complicated. "Sixty-five percent of respondents said their decisions are more complex than just two years ago, and 53% said they face more pressure to explain or justify their decisions."
Decision intelligence (DI) can allow organizations to make quick and optimal decisions. It uses multiple technologies by combining data science, managerial science, and behavioral science with artificial intelligence. DI also visualizes the impact of one decision on several people, processes, and KPIs. The core component of every decision intelligence (DI) solution comprises Artificial Intelligence (AI). AI techniques such as machine learning, deep learning, data visualization, business intelligence, and predictive modeling are vital to an effective DI model.
Now that we've talked about DI and AI, let's also explore the role of BI. Business intelligence (BI) is becoming an integral part of DI solutions as it provides accurate and real-time data and analysis for multiple processes. This helps decision-makers make well-informed, data-driven decisions. BI enables business leaders to analyze and visualize the data with self-service analytics without being dependent on help from the technical staff, making the business process more agile. For example, there's Intelligent Applications, an application of BI, which helps deliver specific analytics and automate several actions when layered on top of data. Such applications offer additional context to business decisions and enhance an organization's ability to use big data lakes for insights on decisions that impact the organization.
So, how do AI and DI connect?
Artificial Intelligence applies to decision Intelligence through technologies like machine learning, predictive modeling, scenario planning, and forecasting. For instance, machine learning models can scan through large data lakes to find patterns and anomalies invisible to the human eye, giving the decision-makers a clear picture of the multiple post-decision scenarios. One such cool application of "ML in decision-making" is the banks' anti-fraud systems that check for any additional user authentication when someone accesses the banking app from a suspicious IP.
Organizations can utilize Predictive Analytics to forecast activity, behavior, and trends by using current and historical data. Predictive modeling uses current and historical statistics to estimate or predict future outcomes. Here's an example of how Predictive Analytics is applied in decision Intelligence: Organizations use this model to identify the reasons for customer attrition. AI techniques can also help build more efficient and effective scenario planning models. Once a set of possible scenarios has been selected, AI can continuously monitor the chosen scenarios and alert the management by suggesting any corrective measures if required.
Similarly, artificial intelligence can be applied to forecasting as well. While organizations have used forecasting models for decades to make informed estimates about the business, AI techniques are now being deployed to improve the efficiency of these models. The main difference between traditional forecasting models and those powered with AI is that the latter can take an unlimited number of factors that influence the forecasts and perform better with vast data. It is pertinent to note that while these tools provide the decision-makers in the organization with the critical insights to make informed decisions, human involvement is also important in making decisions in most cases.
Even though decision intelligence is an emerging field, it has already been applied rapidly in many sectors, such as inventory management, marketing, advertising, banking and financial industry, and healthcare. For instance, let us see how DI is applied in inventory management, marketing, and advertising.
Inventory management: Business organizations can use decision intelligence to optimize different processes in stock management. Decision intelligence can utilize AI-powered demand forecasting models and machine learning algorithms to optimize the inventory levels and stock allocations across the organizational network. These models use current and historical data to predict which product might see increased or decreased demand over a quarter. This can help them decide on the stock volume to be purchased and stored, thereby optimizing the inventory costs. DI models can make highly accurate predictions that could tell the exact number of products to be stored in a specific store at a specific time for higher product turnover.
Organizations can even categorize inventory based on margin, revenue, and product turnover through inventory optimization. This can be done by analyzing historical data on sales. Similarly, decision intelligence can be used to monitor the impact of an inventory management system on profit margins. By detecting any discrepancies in the data, DI models can reduce inventory shrinkage. They can also help companies optimize the price of the products as per their stock levels.
Apart from inventory management, DI can also be applied in warehouse management. Today, warehouses face many challenges such as erratic demand, space constraints, many product variants, and labor issues. These issues impact the entire supply chain and the entities linked to that supply chain. When equipped with data analytics capabilities, DI solutions can utilize the stock and supply chain data to optimize warehousing decisions such as when (timing) and how much (quantity) a product needs to be available in a warehouse.
Marketing: With marketing campaigns becoming increasingly personalized, decision intelligence can be effective for marketers. They can use predictive analytics to offer insights on customized recommendations to the customers. Predictive models can explicitly tell companies which customer segment would prefer a given product and what its best price would be. With this information, marketers can do effective data-driven marketing and merchandising strategies.
In digital marketing, marketers can use DI to study the customers' online behavior and understand their preferences. They can then analyze this data to identify prospective customers and ensure timely and targeted marketing activities for conversion. For example, when sending a discount offer or nudging the customer to check out the product from the cart. DI models with natural language processing can also help in analyzing customer queries and responses to check if the customers are satisfied with the product or service. They can also deduce the possible reasons for customer satisfaction or disappointment. Organizations can use all these insights to take appropriate actions to stop customer attrition. Meanwhile, machine learning can run algorithms on the customer data, identify ideal customers, and create a list of prospective customers for targeted marketing campaigns.
Nagarro and decision intelligence
Decision intelligence is set to become the future of decision-making soon. Organizations can either jump on to the innovator's team to stay ahead of their competitors or lag behind. If you want to be an innovator and are looking for decision intelligence models to address your business needs, we can help. At Nagarro, our team has worked with leading companies in developing and deploying decision intelligence models.
Nagarro recently collaborated with a client to build a decision intelligence solution, where we combined heuristics algorithms and AI optimization. How's this done? We first employ a heuristic algorithm to find a possible sequence of deliveries that satisfies all the constraints. An AI-based algorithm optimizes the series of customers proposed by the heuristic algorithm. The algorithm minimizes the distance traveled and works under time-and-demand constraints. After this, we work on identifying capacity estimation, route plan, and visualization to reduce the total distance traveled and associated carbon emission. In this case, the number of vehicles required decreased from 25 to 18. Similar solutions can be developed for different last-mile delivery space problems.
A lot more came out of our work in this domain, and if you'd like to know more, we'll be happy to share more. It'll be an intelligent decision to work with Nagarro's experts on decision intelligence. Reach out to us by filling out this form.
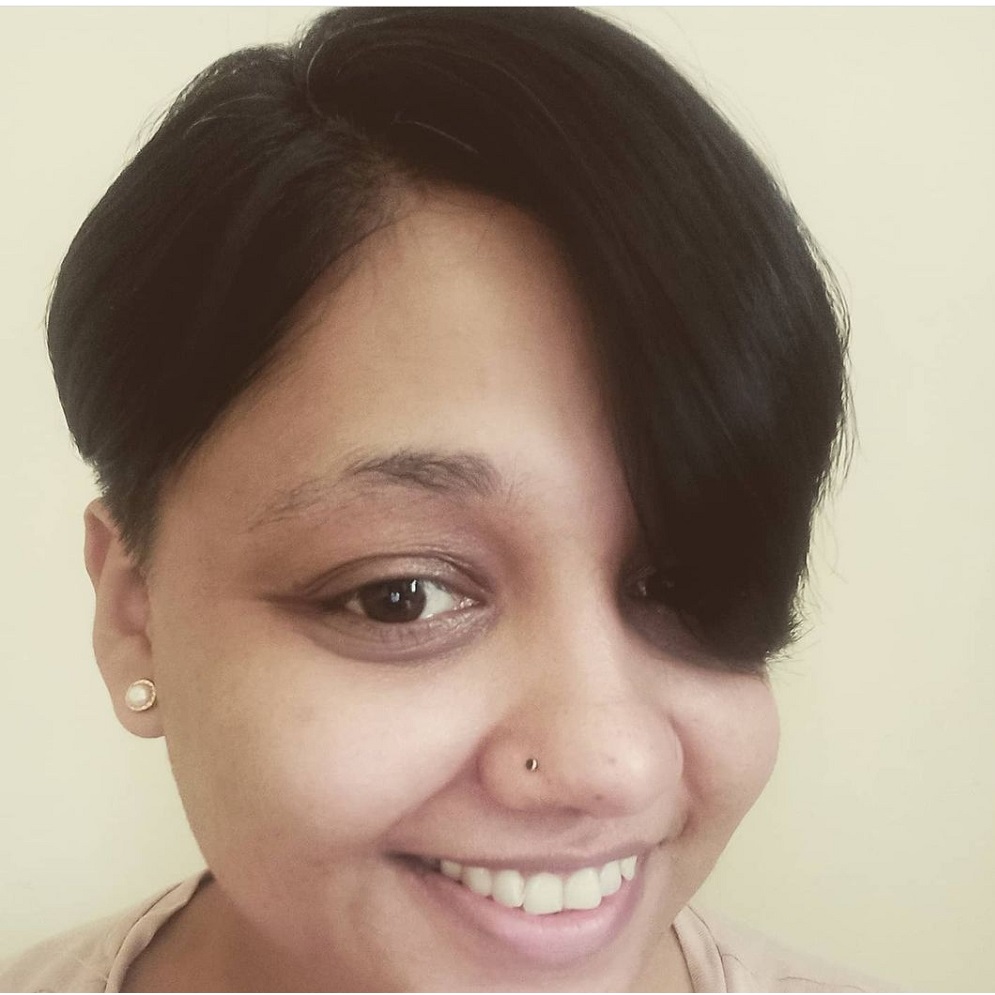
Shubhra Pant
I tell stories.