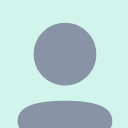
Michel Dorochevsky
Wearable technology or
Nagarro
How
Let’s say you are enrolled in a university course for electrical engineering and simultaneously pursuing a distance learning course to become a technician. You want to start studying through your course material right away. You put on your wearable fitness tracker, connect it to your tablet, and click on the e-learning app. You start with a difficult chapter on resistors connected in series and parallel
Project overview and goals
Motivation is a major factor to facilitate deep learning processes. It makes learning fun and interesting, and as a result improves the overall learning process. If motivational problems are detected early, the learning processes can be modified and the learning content can be adapted to the needs of the student.
The goal of the SensoMot research project is to predict critical motivational conditions using sensor measurements collected by wearable devices. By deriving adaptive mechanisms, the learning process can subsequently be tailored to meet the motivational needs of the student. Based on these objective sensor data (e.g. indicating stress or boredom) algorithms for the learning environment will adjust internal variables like the learning speed, level of difficulty or propose alternate learning paths.
Prototypes of adaptive learning scenarios for a university course on “Nanotechnology” as well as a distance learning course on “Electrical Engineering” will be implemented in the project. These will be integrated and evaluated based on the e-learning and e-testing platform “CBA ItemBuilder”, a product developed by Nagarro for its customer DIPF (German Institute for International Pedagogical Research) which is already in use for many years. The resulting learning systems will be made available to a broad variety of educational applications thereafter.
The innovation around SensoMot will facilitate (for the first time ever) detection of motivational obstacles in the way of learning, thanks to non-obtrusive sensors, and adapt learning content accordingly. Learning motivation increased in this manner could lead to greater learning success and lower dropout rates in a wide range of technology-based learning situations.
Challenges
We are facing three major challenges:
- Can we find wearable devices and provide the “right” sensor data, in a streaming mode to be able to deduce motivation levels? This is not obvious as many of the commercial wearable devices do not provide open interfaces and access to raw data. Typically heart rate, skin resistance, eye tracking and mouse/keyboard movement are used in similar research projects.
- Can we apply adequate pattern recognition and machine learning techniques to infer motivation levels from raw sensor data? The intended process chain is depicted in the diagram below, which shows consolidation of different sensor data via pattern recognition and machine learning to identify motivation indicators and dynamically adapt the learning content accordingly.
- Can we ensure that this sensitive data is secure enough to build the necessary trust so that students are willing to use such a system in their e-learning process?
Outlook
The result of this research could be a milestone for building adaptive learning environments and making learning processes more efficient, particularly in the context of lifelong learning. But even beyond education; for many other applications the ability to detect motivation levels can be envisaged, e.g. to assess the quality of children nursery or aged care work.
Project consortium partners
SensoMot is conducted by a consortium of 6 industrial and research partners and sponsored within the program “Tangible Learning” (“Erfahrbares Lernen”) of the BMFB.
Nagarro GmbH - Munich, leading the consortium, will provide the integration of sensor data collection into the “CBA ItemBuilder” platform and develop the basic mechanisms for adapting the learning content at runtime.
The German Institute for International Educational Research (Deutsches Institut für Internationale Pädagogische Forschung) - DIPF is responsible for the scientific project co-ordination.
The distance learning institute “Fernlehrinstitut Dr. Robert Eckert GmbH” (Eckert) ) is the second commercial consortium member besides Nagarro and will provide the test bed and validation for a distance learning course on “Mechanical Engineering”. It is the natural first user of such a technology.
The “Technical University of Ilmenau” (
The Medical School Hamburg (MSH) will bring in its research competence for the theoretical and empirical identification of motivation indicators and the derivation of adaption algorithms.
The Leuphana University Lüneburg (Leuphana) will provide the machine learning technology to deduce motivation levels from the raw sensor data.
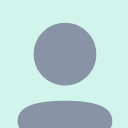