Imagine a trader sifting through stacks of market data, struggling to identify patterns amid rapidly fluctuating prices. Now picture another trader, empowered by generative AI, swiftly identifying hidden trends, predicting market shifts with impressive accuracy, and adjusting strategies instantly. This is no longer a distant possibility—it's today's reality in derivative trading. Generative AI is reshaping the future of derivative trading with new opportunities and trading strategies that can help analyze vast data, predict market trends and optimize strategies in real-time. As traders navigate volatile markets, Gen AI enhances decision-making, boost efficiency and fuels innovation.
Banks and financial institutions recognize this potential, planning to boost their AI investments to over $400 billion by 2027. This massive surge in investment underscores AI transformative impact. Though traditional trading systems stay prevalent, AT-driven technologies are rapidly reshaping derivative markets.
McKinsey highlights that AI quantitative trading systems outperform conventional methods, enabling developers to build applications 56% faster. Patent filings echo this acceleration, with AI-related algorithmic trading innovations showing a steep rise from 19% in 2017 to more than 50% since 2020. The International Monetary Fund further emphasizes that this shift could fundamentally reshape trading ecosystems.
In this article, we will examine why traditional derivative markets are becoming increasingly obsolete and explore how AI setting new benchmarks for trading efficiency and market analysis.
Understanding Generative AI - and why it matters here?
Generative AI isn’t just another tech buzzword. Today, it’s a powerful subset of artificial intelligence that goes beyond analyzing data—it creates! From creating original content, be it be text, realistic images and videos, to even building complex financial data models. This capability makes it a game-changer for industries that require innovation, creativity, and the ability to predict future trends—like derivative trading.
The evolution of Derivative Trading
Derivative trading has always been about strategy—albeit the tools have evolved drastically over time. In the early days, trading was more based on intuition, experience, and basic analytical tools, i.e. charts, etc. Then came the revolutionary electronic trading platforms in the late 20th century, enabling speed and accessibility. But the real transformation began with algorithmic trading, where automation took center stage and rule-based trading strategies became the norm.
Now, we are entering a new era, driven by Gen AI. Unlike past transformations that reinvented the wheel by enhancing the existing process, this one is redefining what’s possible. From smarter trade execution to real-time risk modeling, generative AI is not just enhancing the playbook—it’s rewriting it
How AI algorithms are reshaping derivatives trading
AI algorithms have completely transformed trade execution, analysis, and optimization in the derivatives market. Every part of trading operations is being reimaged by intelligent systems that learn, adapt and predict with unprecedented precision.
1. The dawn of trading algorithms – From rules to learningTraditional rule-based systems worked with rigid rule-based conditions that had to be manually updated to remain effective. Whereas AI-driven algorithms continuously learn from new data, adapts the strategies real-time without human input.
Just look at the numbers–Patent applications for algorithmic trading showed AI content jumped from 19% in 2017 to over 50% by 2020, right about the time Large Language Models (LLMs) appeared, indicating a widespread technological rebuild across the industry.
Machine learning systems now outperform their traditional predecessors by spotting complex, non-linear market patterns in financial data. For instance, it can analyze more data types, including unstructured information from news and social media. They are not just faster, but smarter with the ability to adapt to market changes on the fly without manual intervention. In high-speed derivates markets, this agility is essential for survival in the long run.
2. Up-to-the-minute data analysis capabilitiesWhen it comes to derivative trading, timing is everything. AI systems today can process vast amounts of data in milliseconds and act almost instantly. This speed changes how traders operate.
Let’s take the example of Federal Reserve meeting minutes: AI systems have been analyzing these complex documents with remarkable speed since 2017. The stock price movements seen just 15 seconds after release matched the long-term directions seen after 15 minutes. That means AI is extracting meaningful insights faster than a human analyst could.
These systems don’t just analyze, they rebalance investment portfolios in real-time to increase market liquidity. They identify and respond to fraud within milliseconds. The systems adapt their models continuously without requiring complete retraining.
3. Pattern recognition in market volatilityMarkets move fast and are unpredictable. AI thrives in this volatility, by spotting hidden patterns that human traders or traditional tools might miss. Deep learning models, particularly neural networks, excel at identifying non-linear relationships within historical data, price fluctuations, and trading volumes. This ability turns potential market chaos into structured, useful information.
What’s more? Now AI trading systems tap into unconventional or alternative data sources: from social media sentiment to satellite imagery to global news cycle and create a complete picture of the market dynamics.
The impact is clear - a recent industry survey found that 47% of intermediaries and 48% of end users say market analysis and research have changed most dramatically due to AI.
Four AI technologies leading the charge in derivative markets
The four breakthrough AI technologies brings unique capabilities- each pushing the boundaries of what’s possible in modern day derivative trading.
1. Smart Market Interpretations with Large Language Models (LLMs)Large Language Models (LLMs) like GPT-3 have become powerful tools particularly for market sentiment analysis. With their ability to grasp context and nuance in the financial text (news, reports and documents) better, they outperform traditional classifiers.
A recent study published shows LLMs stock return prediction accuracy of 74.4 %, producing decent results in portfolio management with a Sharpe ratio of 3.05 and generated a 355% investment gain in just two years. By transforming unstructured data into actionable insights, LLMs give traders an edge before the markets even moves.
Neural networks, such as LSTM (Long Short-Term Memory) models are changing the game into price derivatives. Unlike old models with fixed parameters, these models detect hidden patterns in a non-linear way across different time periods - making them ideal for financial forecasting or accurate derivatives pricing.
Scientists found a breakthrough approach. They train neural networks to learn the gap between derivative prices and their asymptotic expansion instead of learning the price directly. The method requires 100 to 1,000 times less training data than direct price learning. That makes advanced pricing strategies accessible even to firms with limited historical data.
3. Smart Trading Strategies with Reinforcement LearningReinforcement Learning (RL) takes trading strategy development to the next level. RL agents learn by trial and error, refining their tactics through simulated market interactions.
For instance, The Soft Actor-Critic (SAC) model outperformed market benchmarks in a down market by:
- Doubling annual returns
- Tripling cumulative returns
- Keeping better Sharpe ratios even in volatile conditions
RL systems can run millions of virtual trades without risking real money and gain decades of experience in hours.
4. Scenario Testing on SteroidsGenerative AI is revolutionizing risk modeling and scenario planning in derivatives markets. Models like Variational autoencoders (VAEs) and denoising diffusion models (DDMs) create synthetic yet synthetic market data that keeps the statistical properties of real markets while generating new scenarios as well as fill in the gaps where historic data falls short.
With these synthetic stress tests, risk managers can now prepare and test strategies against unlikely-yet plausible-market conditions. Thus, trading algorithms become more reliable and resilient to black swan events and extreme market swings.
_____
These four AI technologies explain why old-school derivatives markets can't keep up. AI-powered alternatives offer better speed, accuracy, and adaptability in today's complex digital world.
Key Applications of Gen AI in Derivative Trading
- Predictive Analytics and Market Forecasting
One of the most significant applications of Gen AI in derivative trading is predictive analytics. By analyzing vast volumes of historical and real-time data, Gen AI models uncover subtle patterns and trends that might elude the most seasoned traders. This capability translates into capable decision-making and fewer cost missteps.
📈
According to a report by Accenture, 75% of financial institutions are investing in AI-driven predictive analytics to enhance their trading strategies.
- Risk Mitigation and Scenario Simulation
Risk is the name of the game when it comes to derivative trading. And Gen AI brings a vital value in this playbook. AI models help identify potential risks and formulate dynamic mitigation strategies. For example, AI models can simulate various market scenarios and predict the impact of different trading strategies, allowing traders to make more informed decisions.
💡
AI-driven risk engines that auto-suggest hedging strategies based on live data feeds.
- Algorithmic Trading and Strategy Optimization
When algorithmic trading has been a cornerstone of modern derivative trading for so long, Gen AI only elevates it. Besides executing predefined strategies, it continuously optimizes these strategies based on real-time market conditions for significantly better outcomes.
📈
A study by JP Morgan found that AI-optimized trading strategies outperformed traditional strategies by 15% in 2022.
- Sentiment Analysis with Natural Language Processing (NLP)
Narratives and not numbers drive markets. Gen AI with Natural Language Processing (NLP) capabilities can analyze news articles, social media posts, and other textual data to gauge market sentiments- and translate that into invaluable and actionable trading signals.
💡
Sentiment analysis tools that provide real-time sentiment scores for specific assets, allowing traders to adjust their strategies accordingly.
- Synthetic Data Generation for Model Training
Availability of high-quality data is a cornerstone for effective AI, but real-world financial data can often be incomplete, censored, or limited. Gen AI addresses this by generating synthetic data that mimics real-world market conditions, providing a rich dataset for training more accurate and robust models.
📈
Gartner predicts that by 2025, 60% of the data used for AI training will be synthetically generated.
Innovative ideas for Gen AI in Derivative Trading
AI-Driven Portfolio Optimization
Gen AI can be used to optimize trading portfolios by continuously analyzing market conditions and adjusting the portfolio composition to maximize returns and minimize risk.
Real-Time Fraud Detection
Fraud is a significant concern in derivative trading. Gen AI can be used to detect fraudulent activities in real-time, allowing for immediate action to be taken.
📈
A report by PwC found that 45% of financial institutions have experienced fraud in the past two years, highlighting the need for advanced detection mechanisms.
Personalized Trading Assistants
GenAI can be used to create personalized trading assistants that provide tailored advice and recommendations based on an individual trader's preferences and risk tolerance.
Blockchain Integration for Smart Contracts
Blockchain technology can be integrated with GenAI to create smart contracts that automatically execute trades based on predefined conditions, reducing the need for manual intervention.
📈
According to a report by MarketsandMarkets, the blockchain market in financial services is expected to grow from 1.5 billion in 2021 to 22.5 billion by 2026.
Why traditional trading systems can't compete
As AI reshapes the financial landscape, traditional trading methods are falling behind, being nowhere near as effective, as quick or as sophisticated. Here’s why traditional trading systems can’t compete.
- Human vs Machine – The speed limitations
The speed factor doesn't just give AI an edge—it changes everything. AI not just acts fast; it acts in microseconds. In high-frequency trading environments, every second count and AI can identify and capitalize on fleeting price differences before human traders can spot them.
Besides reaction time, there is another challenge: decision fatigue. Traders make hundreds of decisions daily — as hours pass, decision-making performance drops. Decision fatigue leads to emotional trading, overconfidence, and bias. AI systems, however, perform consistently well whatever the time of day or market conditions. Loss-aversion and confirmation bias trick traders into holding losing positions too long or reading market signals wrong.
- Overwhelming volumes of datasets
Markets create massive data volumes each second —price movement, global news, economic indicators, social media trends and more. No person can watch and analyze all important market information at once. AI systems thrive in this chaos. They can analyze millions of data points instantly and spot subtle insights invisible to human traders. In the complex world of derivative markets, this level of accuracy is not a luxury but a must.
- Limited pattern recognition capabilities
Human analysts can spot the obvious trend, but AI-powered systems can find the invisible. To name just one example:
- Neural networks spot non-linear relationships that traditional methods overlook
- Machine learning models see subtle connections between seemingly unrelated markets
- AI systems find market inefficiencies by analyzing patterns across different timeframes
Most traditional models heavily rely on historical data, replaying old data at high speed instead of modeling the why behind the major market events. AI technologies break that mold by learning evolving patterns and not just repeating old ones.
The role of technology partners in enabling Gen AI in trading
Behind every AI-powered trading system, there is a robust tech infrastructure that enables firms to harness complex algorithms and vast data sets. IT companies are critical enablers of this transformation. These strategic partnerships enhance operational efficiency, allowing traders to execute transactions with speed and accuracy, streamline processes, minimizing latency during trades.
Moreover, tech partners go beyond technology and bring specialized domain knowledge, and expertise in regulatory compliance ensures that financial institutions navigate the evolving landscape of rules and standards seamlessly.
- High-Performance Infrastructure & Cloud Platforms
The computing power required for Gen AI is immense. IT companies are critical facilitators in providing the necessary scalable infrastructure, including high-performance computing resources and cloud-based platforms.
- Data Management and Integration
With data flowing from multiple sources and channels, seamless data integration becomes key. Tech partners enable unified data management frameworks that feed GenAI engines with clean, connected, and enriched data.
📈
According to IDC, 70% of organizations are investing in data management solutions to support their AI initiatives.
- Security and Compliance
When it comes to trading, data is everything. Thus, security and compliance is non-negotiable. IT companies offer solutions that ensure data security and regulatory compliance.
- Custom AI Solutions and Consulting
Every financial institution has unique needs and challenges. IT companies provide custom AI solutions and consulting services, helping organizations tailor Gen AI applications to their specific requirements.
📈
A survey by Deloitte found that 60% of financial institutions are working with IT consultants to develop customized AI solutions.
AI quantitative trading: The new market standard
AI-powered systems are becoming the new measure in quantitative trading. AI-driven quant strategies now make up over 40% of hedge fund trading volumes in 2024. This shows a clear move in market dynamics.
How quant models utilize AI for superior returns
AI quantitative models perform better because they know how to extract meaningful insights from complex datasets that were too difficult to process before. These systems spot subtle patterns across multi-dimensional data and find mispriced assets and market inefficiencies that conventional approaches miss.
The difference in performance stands out. Next-generation AI approaches beat market indices by 1.5-2%, while traditional quant models deliver around 1%. This extra alpha creates substantial value for investors over decades.
Machine learning models understand relationships between multiple signals better than traditional models. These systems don't just weight predefined signals, they adapt to changing market conditions and learn complex interactions between hundreds of variables.
Case study: AI-powered hedge fund performance
Renaissance Technologies shows how AI disrupts hedge fund performance. Their Medallion Fund uses sophisticated machine learning algorithms to analyze complex market data. The fund consistently generates exceptional returns by finding patterns humans can't detect.
High-Flyer, a Chinese quantitative hedge fund, shows how deep AI integration creates adaptability in volatile markets. Their strategy combines algorithmic trading, AI-driven stock selection, and complete investment approaches that match international powerhouses.
Traditional institutional investors are seeing remarkable results too. The Teacher Retirement System of Texas reports "tremendous" efficiency gains from using AI-based decision trees for equity portfolio management. They achieved this with open-source tools, which makes it easier for others to enter the market.
Integration of alternative data sources
AI quantitative trading has changed how we utilize data. Modern systems analyze more than just financial statements:
- News sentiment and social media activity (2+ million articles analyzed annually by some funds)
- Patent filings (50+ million globally per year)
- Geospatial data and satellite imagery
- Consumer transaction information
Alternative data integration proves its worth—72% of investment firms report better signals from these sources. More than a fifth credit over 20% of their alpha generation directly to alternative data.
All the same, challenges exist. Firms using alternative data worry about data ownership issues (36%), security risks, compliance burdens, and the potential risks of acquiring material non-public information (all at 33%).
Regulatory challenges in AI-driven markets
AI continues to reshape financial markets while regulators worldwide face unprecedented challenges in oversight. The state-of-the-art technology's rapid rise has created gaps between innovation and regulation. This raises fundamental questions about market integrity and how to protect investors.
- Current regulatory frameworks
Different jurisdictions handle AI trading differently based on their priorities and philosophies. Most financial regulatory frameworks stay technology-agnostic and principles-based. These frameworks can theoretically catch harmful AI behaviors no matter what technology powers them. The EU leads the pack with its groundbreaking AI Act that puts systems into risk levels and demands strict requirements for high-risk financial tools.
The UK's financial regulatory system covers market manipulation by AI systems, as stated in Recital 38 of the UK Market Abuse Regulation. This comes as good news despite market concerns. The US lacks detailed federal legislation that specifically regulates AI development. However, Congress is looking at more than 120 AI bills right now.
- Transparency issues with black-box algorithms
Sophisticated AI trading systems work like black boxes, which creates troubling challenges. Developers often cannot explain the reasoning behind machine learning models, especially those using reinforcement learning. Regulators find it hard to check if these opaque systems follow existing rules.
Market abuse regulations use the idea of "reasonable suspicion," but this becomes tricky with AI-driven trading. Companies struggle to tell the difference between legitimate AI trading strategies and potential abuse. The risk grows because a few third parties control most critical AI services, creating dangerous dependencies.
- Proposed solutions for AI oversight
Regulators now take multiple approaches to make AI more accountable. Some want bigger penalties for those who use AI technologies to commit fraud or manipulate markets. Others say future oversight should focus on human developers and data regulation.
Many jurisdictions now require documentation, testing, and regular audits to check compliance. The CFTC wants feedback about AI in derivatives markets. They need input about definitions, use cases and risks before taking regulatory action.
Good oversight needs both transparency and innovation to work together. Explainable AI (XAI) and open-source models offer ways to solve the black box problem. These approaches help maintain technological progress.
Challenges and ethical considerations
While the potential benefits of Gen AI in derivative trading are immense, there are also significant challenges and ethical considerations that need to be addressed. These include issues related to data privacy, algorithmic bias, and the potential for market manipulation.
💡
Ethical AI frameworks that ensure transparency, fairness, and accountability in AI-driven trading systems.
Prospects for Derivative Trading with AI
The integration of AI into derivative trading is poised to revolutionize the industry by enhancing market efficiency, reducing risk, and improving decision-making. By 2025, AI-driven trading algorithms will further evolve, offering real-time portfolio optimization, automated risk assessment, and data-driven strategies that minimize human biases. AI empowers retail and institutional traders to make more informed decisions by rapidly analyzing vast data and uncovering patterns undetectable by human analysis.
The IMF’s Global Financial Stability Report highlights that AI-based trading platforms effectively reduce impulsive decision-making and strengthen traders' risk management capabilities. Nonetheless, challenges remain, including data security concerns and potential market volatility triggered by algorithmic trading behaviors, as seen during the 2020 market disruptions. To secure sustained success, financial institutions must balance AI-driven analytics with human oversight and adherence to regulatory compliance measures to prevent excessive dependence on automated models.
Predictions for the Future of AI-Driven Trading
Looking ahead, AI-driven trading is expected to become more autonomous and sophisticated. Let’s look at some of the upcoming trends.
- By 2030, AI will become central to real-time investment decision-making. Robo-advisors will deliver personalized portfolio recommendations derived from extensive data analysis. Enhanced AI-powered risk assessment models will enable financial institutions to proactively detect and mitigate risks, fostering a more stable and predictable trading environment.
- AI adoption in financial services will rapidly accelerate in emerging markets, where fintech firms leverage AI to enhance financial inclusion and expand access to sophisticated investment opportunities.
- By 2050, AI may fully automate trading processes. Autonomous banking systems will manage investment, execute trades, and optimize portfolios without human intervention. This transition will likely be accompanied by blockchain-based AI solutions to ensure transaction security, transparency, and efficiency.
As AI continues to dominate trading and financial decision-making, ensuring ethical AI deployment, regulatory oversight, and cybersecurity measures will be essential to preserve market stability and investor trust.
Conclusion
Generative AI is set to transform derivative trading with unprecedented capabilities in predictive analytics, risk management, and strategy optimization. IT companies will play a crucial role in enabling these advancements, providing the necessary infrastructure, data management solutions, and security measures.
Numbers tell a compelling story.
- AI quantitative models yield 1.5-2% higher returns than conventional strategies.
- Large language models predict market movements with 74% accuracy. Neural networks have altered the map of derivatives pricing.
- Financial institutions will double their AI investments to $400 billion by 2027.
As the financial industry evolves, addressing the associated challenges and ethical considerations associated with AI deployment is vital to ensuring responsible and sustainable outcomes. Machine learning algorithms can process and act upon massive data sets in mere milliseconds, adapting swiftly to market fluctuations—capabilities essential for maintaining a competitive edge. Regulatory frameworks need refinement, particularly for AI systems' transparency and oversight.
Traders and institutions must accept new ideas to stay competitive. Their success will depend on implementing these powerful tools while navigating the changing regulatory environment effectively.
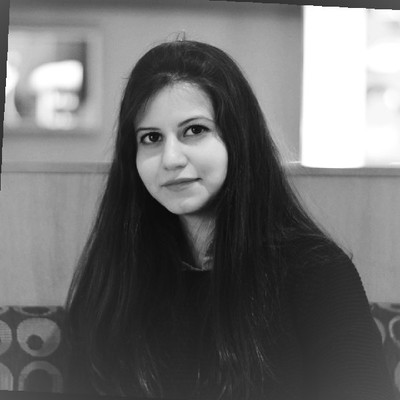