To comprehend the significance of analytics in the automotive sector, it is crucial to grasp the ongoing disruption within the sector marked by several defining factors, such as changing mobility preferences and the emergence of new technologies.
According to McKinsey, “the levels of disruption in the automotive industry coming over the next dozen years are likely to exceed those of the previous 50 or more.” Its ACES framework underlines four pillars supporting the evolution – autonomous driving, connectivity, electrification, and shared mobility.
On the one hand, the demand for autonomous vehicles is pushing OEMs to redefine their product strategy, and on the other hand, electric vehicles are seeing increased demand. Meanwhile, shared mobility and app-based transport are changing mobility consumption patterns. And all these factors are influenced by the connectivity changes across countries.
As the auto sector witnesses its biggest evolution yet, data science, analytics, and artificial intelligence can help OEMs keep up with the changing landscape and act as a differentiator by delivering value across functions, especially marketing, sales, and operations. This article looks at how analytics delivers value in these areas.
*We are not separating data, data science, and AI but using analytics as an umbrella term for all these technologies.
Data, analytics, and decisioning systems
Before diving into how analytics can deliver value for auto OEMs, let us demystify decision systems, data, and analytics. A decision system is a software that enables business users to look at data from multiple sources and extract insights using analytics to make informed decisions. Data is at the root of this ecosystem. Organizations utilize data to formulate the right questions and then answer those questions through analytics.
Data, analytics, and decisioning systems are not just interrelated, but they are also evolving simultaneously. Data volume is constantly growing, and the decisioning system are also moving from passive to automated systems. Meanwhile, analytics is also evolving.
In an automotive context, decisioning systems are gradually evolving from passive to automated. That doesn’t mean that all passive systems become augmented and all augmented systems become automated.
How OEMs can leverage analytics for automotive marketing
A study suggests that 90% of people research online before visiting the showroom when buying a car. On average, a customer spends 14 hours online researching different models and specifications, and two-thirds of them make a decision online. D2C platforms such as Carvana and Amazon Vehicles are gradually eliminating traditional car dealerships.
There is no one-size-fits-all when it comes to marketing. As a chief marketing officer, how do you distribute your marketing budget? How do you decide where to invest, which customers to focus on, and which channels to choose? What is the basis for that decision-making? Are you using any data-driven approaches? Are you using analytics while making these decisions?
Marketers must address the following for a well-crafted strategy:
- Who should you target
- Where should you run your campaigns
- What should the ad creative look like
- When do play marketing interventions
Organizations can use different analytics-driven approaches to identify the right customers, choose the right channel and analyze the impact of their campaigns. Causal and incremental modelling help identify the right content for a campaign, whereas programmatic advertising and attribution modelling help select the right channels and assess a channel’s performance respectively. Incremental return on advertising spends helps assess the performance of marketing campaigns.
How automakers can use analytics to achieve better sales
Buying a car can be stressful and tedious for consumers as it involves intense research, price haggling, and navigating complex configurations and offers. At the same time, the sales team is also stressed due to downsizing, ongoing campaigns, sales targets, and pressure to upsell or cross-sell. Analytics can help in the following stages of the sales process:
Lead management: There are three stages of lead management. Lead generation is followed by lead qualification and, finally, lead conversion. The first two involve identifying and assessing leads, whereas the third involves converting a lead. Modeling and analytics can fully automate all three stages of the lead management process.
While working with an automotive company in the Middle East, we discovered they lost 80% of their lead funnel. But most of their sources (80%) worked on these 80% leads instead of focusing on the 20% leads with a much higher probable conversion rate. If they reversed the resource allocation, they could increase their lead conversions to approximately 15 cars daily.
The only reason we made this discovery was data analytics. We looked at all the signals, measured them with outcomes, and built regression models for suggestions. So instead of selling to everybody, companies should invest resources in the leads most likely to convert. And then assess the efforts required to convert those leads.
Cross-selling and up-selling: Cross-selling and upselling require an in-depth knowledge of the customers and the critical predictors beforehand. Organizations need to know which service/product a customer prefers so that they don’t sell them everything with the hope that something sticks. OEMs possess essential customer data, which they can leverage using analytics to cull insights for successful upselling and cross-selling.
Dynamic pricing: Prices are not static anymore. Airlines and hotels have perfected the dynamic pricing model. And now, product companies are equally interested in dynamic pricing models. However, dynamic pricing requires assessing the customer interest in a particular product, which depends on inventory management. It requires organizations to ask questions like how much inventory they can manage. How well do they understand inventory? How well do they understand the customer? Advanced analytics solutions can help answer these questions and assess customer interest in a product.
Streamlining automotive operations with analytics
Post sales, OEMs go through significant processing volume for vehicle delivery, configuration, service scheduling, inspection, and verification of leases/user cars. For efficient operations, they must understand the market trends and demand for specific vehicles and models at the dealership or regional level. Building simple forecasting models and improving them over time is much more effective than conducting surveys.
Machine learning and data science can help OEMs build models that can understand thousands of SKUs and create accurate forecasting models. Organizations no longer need to dedicate resources with in-depth knowledge of an SKU. They can use forecasting tools, and human resources can invest their time in more strategic tasks instead.
Building operationalizations for vehicle inspections and resale value calculations helps OEMs chart the post-sales strategy. While working with a client, we learned how implementing resale value calculations, and AI estimations can be extremely helpful in generating valuable and interesting data.
Automakers can use analytics applications such as customer service bots and document processing automation for processing requirements. Customer service bots that understand the context of service can enhance customer experience. Meanwhile, document processing automation (and other hyper-automation use cases) can significantly streamline the documentation process during a car purchase reducing the consumer’s stress and improving efficiency.
Operationalizing analytics in three simple steps
As analytics becomes a differentiator in marketing, sales, and operations, it will bring value through improved customer experience, higher sales, and lower costs. OEMs can apply analytics within their organizations with three simple steps – discovery, piloting and scaling. They can begin by identifying the areas for analytics intervention and understanding how analytics can bring value to these areas. Follow this with launching pilot projects that can be scaled depending on their success. A methodical approach and an experienced technology partner significantly increase the success rate of any analytics project.
Automotive, Analytics, Ai, Big data and analytics, Transform, Innovate
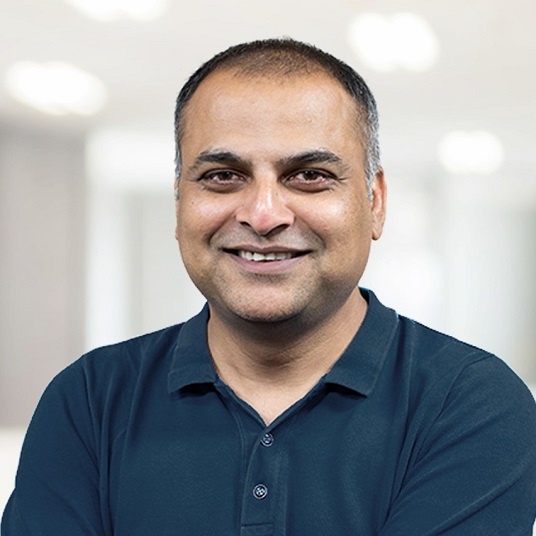
Anurag Sahay
Nagarro
Automotive, Analytics, Ai, Big data and analytics, Transform, Innovate