A customer review is nothing less than a gem for a business. Analyzing these reviews helps understand the customer’s requirements and improve the service or product accordingly. A customer can provide a review (mostly textual) through different mediums, like writing their experience in feedback forms or through social media and e-mail. Analysis of these unstructured text reviews to derive meaningful insights is generally termed opinion mining.
A smart opinion-mining solution can help businesses become customer-centric and place their customers at the heart of everything they do. It is about listening to their customers, understanding their voices, analyzing their feedback, and learning more about customer experiences and their expectations for products or services.
What is Deep Opinion Extraction?
Deep Opinion Extraction is a Nagarro opinion mining solution that uses Advanced AI and NLP approaches to extract domain-specific insights from customers’ textual feedback and produces an aggregate view of feedback across different dimensions with the help of an analytics dashboard.
Consider the below statement as an example to understand it further:
"The food served was great."
If we go with a conventional approach of sentiment analysis (SA), the output will be a positive review w.r.t the aspect “food” (sentiment target). However, many reviews can include multiple aspects, each with its sentiment polarity (positive, negative, neutral). For example, in the following restaurant review:
"The food was tasty, but the services were bad."
The overall SA output of positive/negative does not show the complete picture of the review. The "food" has a positive polarity while "service" has a negative one.
Simply assigning positive or negative polarity hides the user’s actual sentiment or opinion towards a particular aspect. This can be solved by using deep opinion extraction by calculating the sentiment polarity of each aspect separately. We can also take it one step further and find out the words that resulted in these polarities, like "tasty" reflecting positive sentiment and "bad" being responsible for negative sentiment.
Nagarro’s deep opinion extraction aims at this kind of finer analysis; it requires certain aspects of an entity in question, and the sentiment would be classified concerning each of them. Its result gives opinions and sentiments on different entities' features or aspects.
Our opinion-mining approach
There are many challenges in performing these tasks, as identifying aspects and the corresponding polarities are domain-specific, so different domains have different aspects and opinion terms.
Consider the case with domains: Hotel and Restaurant. For the domain hotel, we might consider aspects such as room, cleanliness, and parking while for the restaurant, aspects like food, ambiance, and menu would be more useful. Opinion words may convey different polarities across domains as well. For example, the word “Hot” in the restaurant (meal) domain will have a positive connotation, while it may be negatively connoted in the airline or hotel domain.
We have broken down this problem into the following three subtasks:
- Aspect term extraction (ATE)
- Aspect polarity classification (APC)
- Aspect opinion extraction (AOE)
Aspect term extraction (ATE)
This subtask is to identify the aspects of given target entities using Named Entity Recognition (NER).
It identifies “entities” like food and ambiance from our reviews.
For example, in the following restaurant review:
“The main course served was delicious, but the dessert was disappointing. The ambiance of the place was good as well.”
The highlighted words are the “entities” output from NER, and the entity type of each entity can be:
Entity | Entity Type |
Main course | Meal |
Dessert | Meal |
Ambiance | Ambiance |
Aspect Polarity Classification (APC)
APC or Aspect-based sentiment analysis (ABSA) takes the input of the aspect words and identifies the sentiment polarity at the aspect/Entity level.
“The main course served was delicious, but the dessert was disappointing. The ambiance of the place was good as well.”
For the above NER example, APC outputs can be represented as follows:
Entity | Entity Type | Sentiment |
Main course | Meal | Positive |
Dessert | Meal | Negative |
Ambiance | Ambiance | Positive |
Aspect opinion extraction (AOE)
We used the target-oriented word extraction (TOWE) algorithm to extract the corresponding opinion words for a given opinion target/aspect in a sentence.
Consider the following example:
"My friends and I were on vacation in NY and were referred to Chance by a friend. I found the food outstanding, particularly the salmon dish I had. I also ordered the Change Mojito, which was out of this world. Overall, excellent restaurant!"
Extracted Target | Opinion Words |
Food | Outstanding |
Salmon dish | Outstanding |
Change Mojito | Out of this world |
Restaurant | Excellent |
Results
From the feedback, we have aspects/entities, their respective sentiments, and the opinions expressed about them. We can collocate all the information as shown below from the example:
“The main course served was delicious, but the dessert was disappointing. The ambiance of the place was good as well.”
Entity | Entity Type | Opinion Words | Sentiment |
Main course | Meal | Delicious | Positive |
Dessert | Meal | Disappointing | Negative |
Ambiance | Ambiance | Good | Positive |
Deriving analytics from custom dashboards
The responses from the solution can be used to create interesting analytics and get valuable insights. Let’s analyze the customer reviews of a particular airline through the below-customized dashboards that also reflect the actionable insights.
Figure 1: Custom dashboard for deep opinion extraction for an airline
Figure 2: Countrywise sentiment analysis via custom dashboard
Figure 3: Custom dashboard displaying category-wise sentiment distribution
We can analyze sentiment at a higher level over categories, like in Figure 3; we can infer from the analysis that the “Inflight-wifi” is skewed towards more negative reviews, and businesses can immediately know that it’s an area of improvement.
Such customized dashboards and deep opinion extraction for huge volumes of data will help businesses analyze feedback and investigate gaps quickly and efficiently. Organizations can then come up with calculated efforts in the right business areas for better productivity and profits.
Advantages of deep opinion extraction
Nagarro’s deep opinion extraction solution helps organizations derive a host of benefits, such as:
- Improving data-driven decision-making using aggregated analytics
- Gaining feedback on past activities by analyzing the sentiment flow of different aspects
- Improving customer experience by prioritizing customer pain points with precise accuracy
- Reducing customer churn by developing empathetic communication and engaging with relevant messaging
Technologies applied
Nagarro's Deep Opinion Extraction solution uses an amalgamation of technologies to deliver the very best as per different organizations. Our data science experts leverage:
- TensorFlow/PyTorch
- NER, entity-level sentiment analysis, entity-level opinion analysis
- GCP, cloud functions, cloud run, cloud SQL
- Apache Superset
- Kubernetes/Docker
Conclusion
Deep opinion extraction can help bring more insights, optimize operations, reduce manual efforts, and improve customer experience.
Nagarro provides consultation to ensure that these AI/ML initiatives transition from idea to proof of concept (POC) to the production-ready stage. With a dedicated team that continuously builds smart, intelligent solutions & accelerators, we can help you kickstart your advanced analytics journey. Let’s get together to understand your customers better!
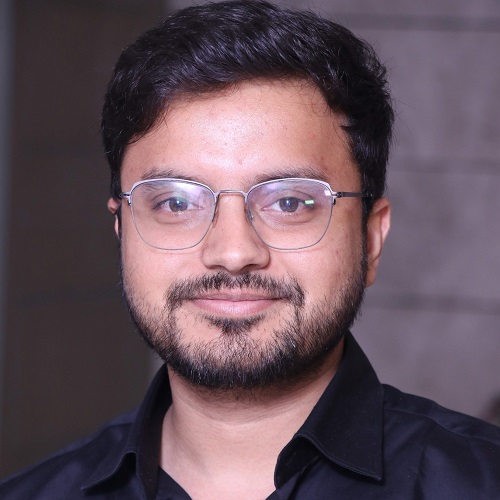